This post was written by Matthew Varona, who spent his internship with the data team building tools and diving into frontliner mobility services during the community quarantine period.
Introduction
Metro Manila was placed under Enhanced Community Quarantine (ECQ) from March 15 to May 31 in response to the COVID-19 pandemic. During this period, public transport was suspended. Transportation for medical frontliners was instead provided by city governments, DOTr, and the OVP. Were these routes enough to cover transport demand during ECQ? As part of my Sakay internship project for Summer 2020, I visualized transit area coverage and demand during April ECQ to find out!
Visualizations
For this study I'll be working with data from April 2020, because quarantine policies generally stayed the same throughout the whole month.
Since public transport was suspended during ECQ, different bodies like LGUs and government offices provided their own routes for medical frontliners. There were slight changes in transit stop availability throughout April, as different agencies reopened or discontinued routes.
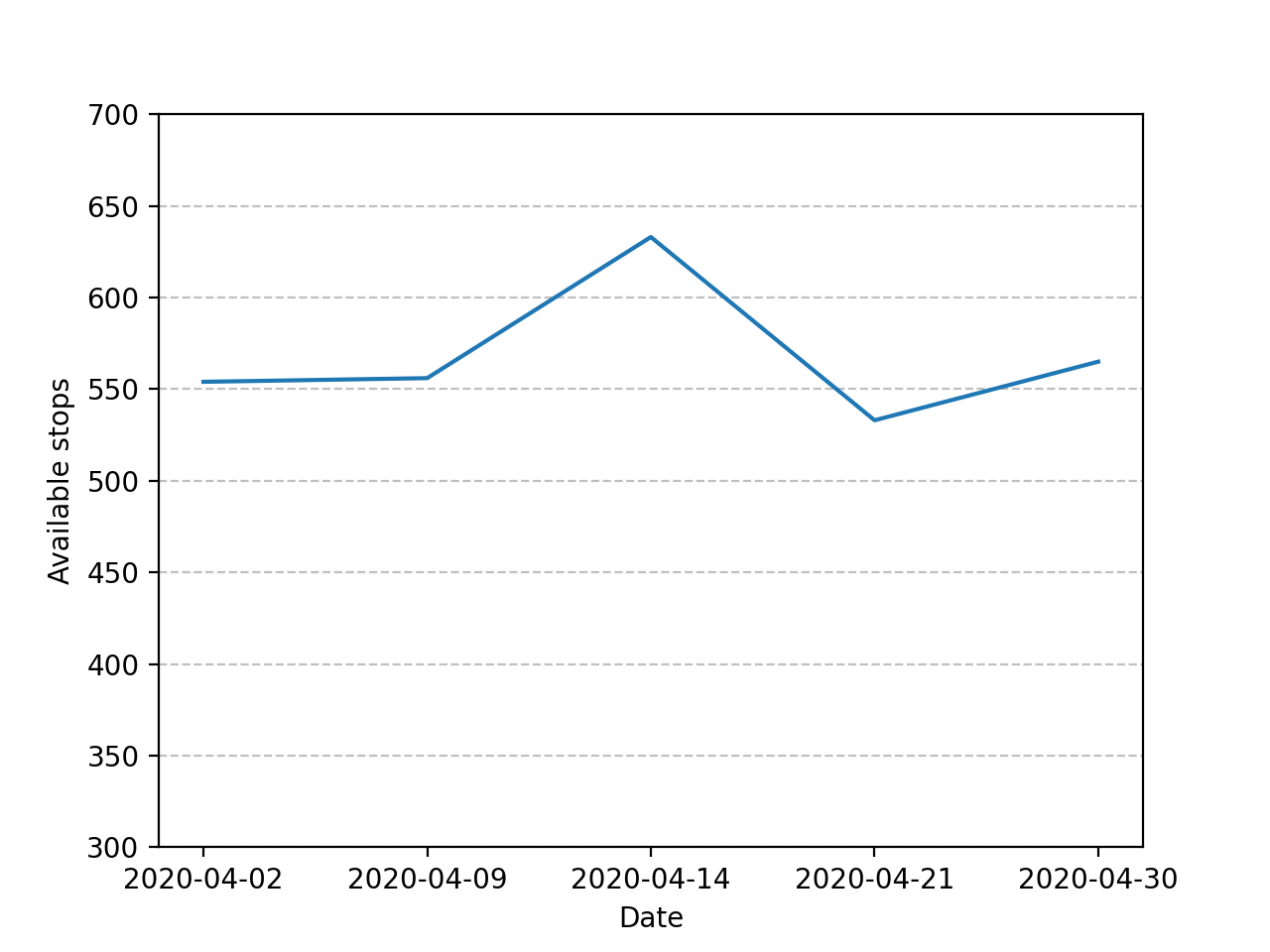
A transit stop's "coverage" can be determined by how accessible it is on foot. Ideally, a stop should be accessible within 400-1000m of walking. For this study, I mapped coverage within 400m and 800m.
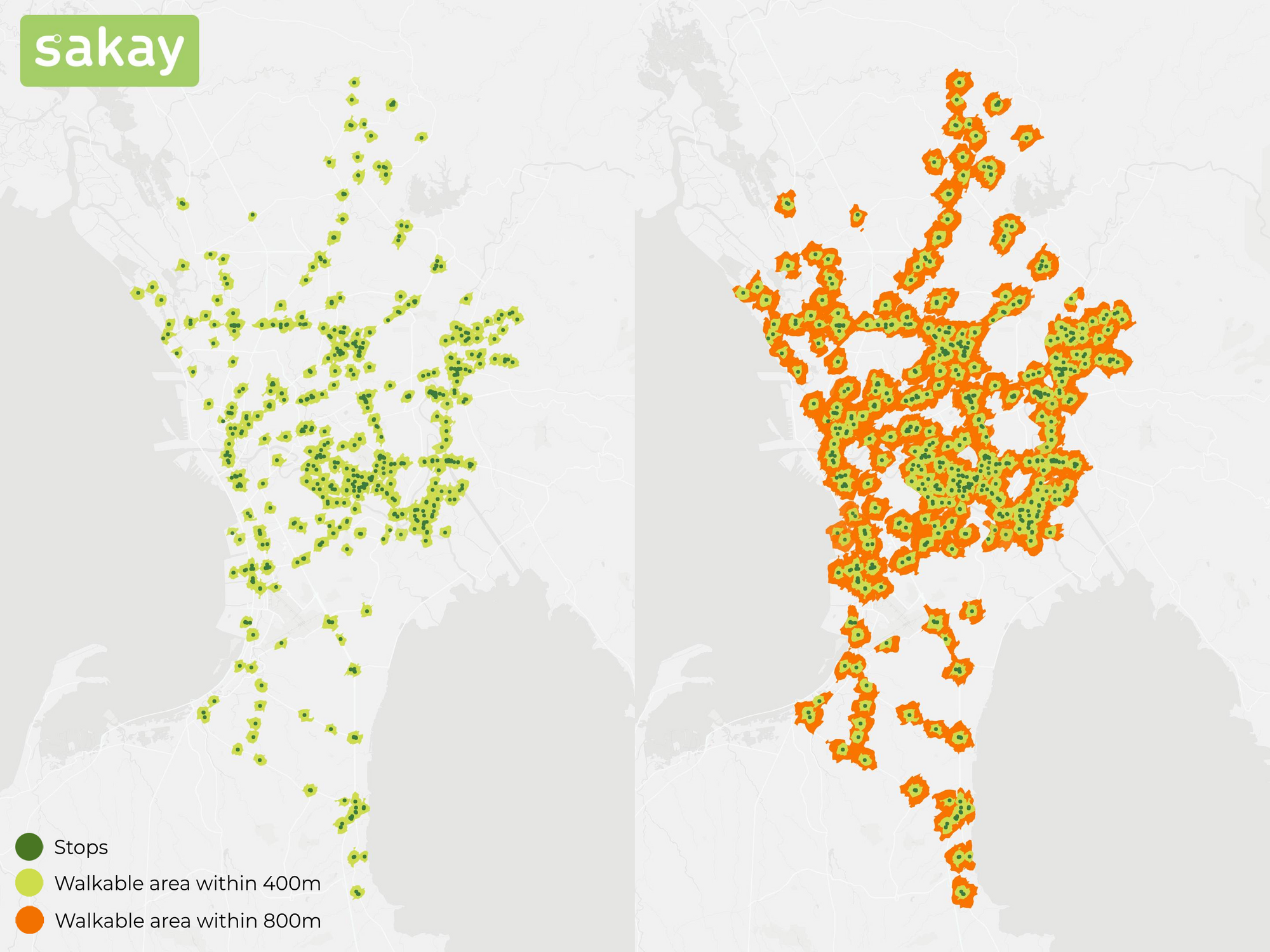
Using April 14 as a baseline – when the most stops were available during April – we can see quite a few gaps in coverage. Taguig, Parañaque, Valenzuela, and parts of Quezon City (Pasong Tamo, Tandang Sora, Katipunan area) all have large areas without a public transport stop within walking distance.
It's worth noting that the coverage presented in these maps are conservative estimates. These visualizations can't really account for buses stopping in the middle of routes, at places other than official bus stops. These informal stops are difficult to map because of factors that cannot be captured by the model (like sidewalk barriers or no-loading-and-unloading signs), or if the stops simply aren't safe places to stop at. Thus, it's possible that a few areas that appear uncovered in the map might actually have access to informal transit stops.
How does this coverage match up against demand for transport? To measure demand, I pulled data on searches conducted on the Sakay app. Searches on the Sakay app are useful data for transport demand because they can tell us where people are coming from and where they want to commute to. I pulled search traffic data from April 2020, as well as April 2019 for a pre-ECQ comparison. Below are heatmaps of search origins (left) and destinations (right):
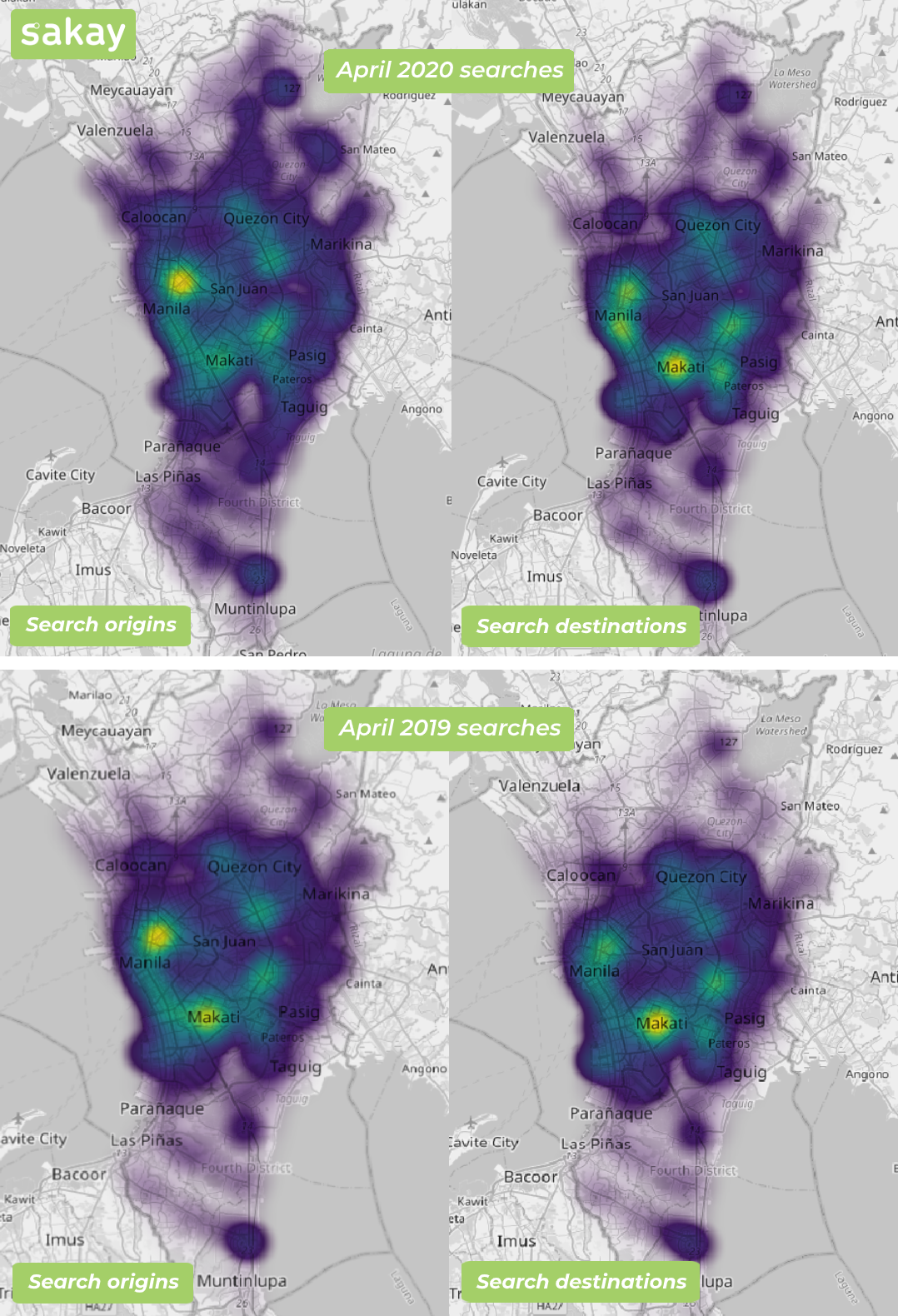
Let's look at April 2020 transport demand first. While the highest concentration of searches falls within covered areas, such as Manila, Makati, and southern Quezon City, there's still quite a lot of demand for transportation outside covered areas. In particular, you'll notice that there's a decent amount of search volume coming from Taguig and central Quezon City, even though coverage is sparser in those areas.
Comparing April 2020 to April 2019, the differences in search patterns aren't drastic. However, we can notice that search traffic in April 2020 was more widely dispersed throughout different parts of Metro Manila. In comparison, April 2019 had more concentrated searches in work hubs like Manila and Makati. With work-from-home becoming the norm for many office jobs, it makes sense that less people are trying to travel to office-dense areas.
There were also significantly more searches conducted in April 2019 when there was no lockdown, although this isn't reflected in the heatmaps above which show search density rather than volume.
Diving deeper into coverage, we can see what percentage of searches fell within 400m of stops:
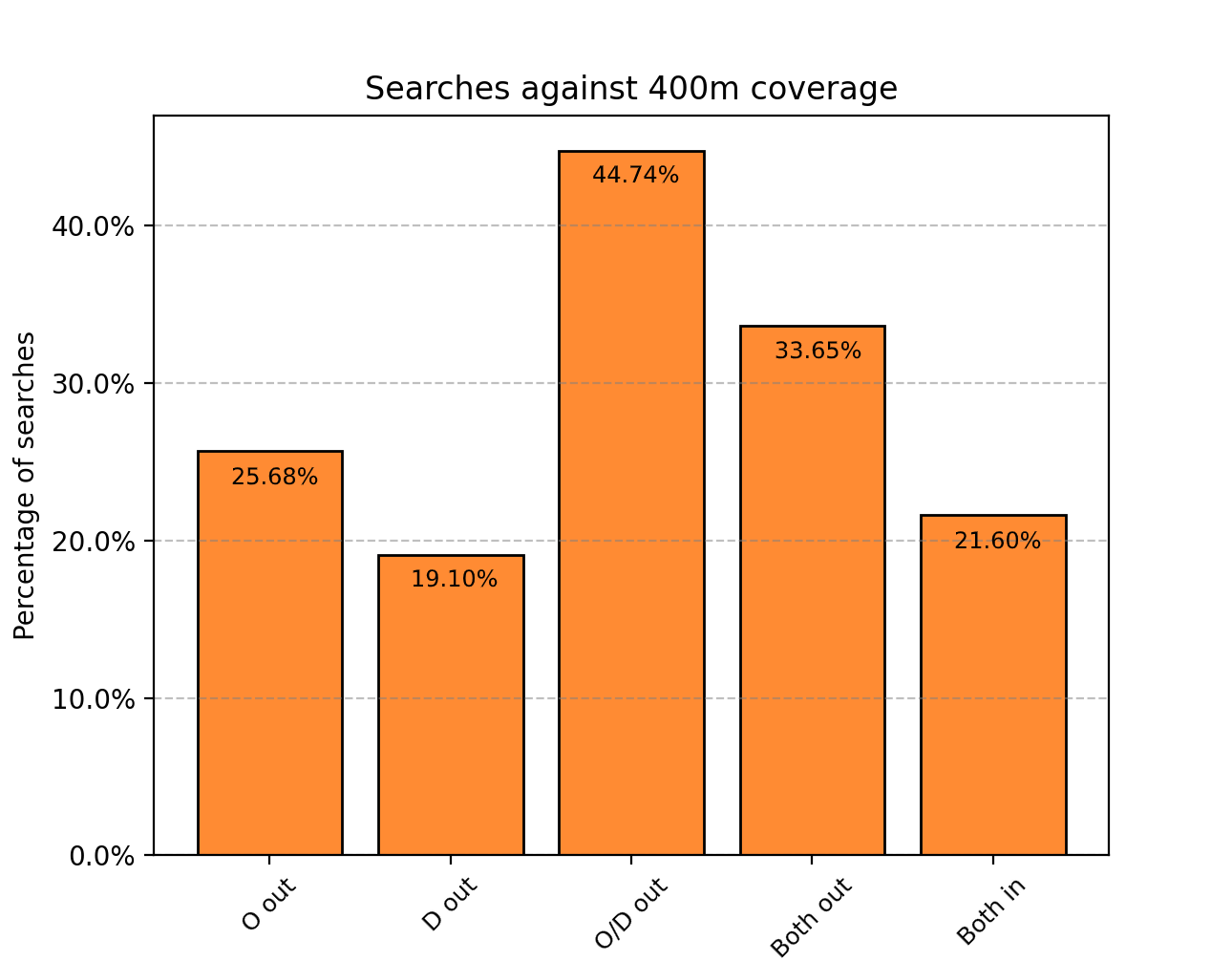
Note that O/D out refers to searches with either the origin or destination outside of coverage, but not both.
From the graph, we can see that just over 20% of searches fully fall within coverage. Around 45% have either origins or destinations outside of coverage, and nearly 35% completely fall outside the coverage area. Essentially, up to 80% of commuters would either have been unable to reach their destinations, or would have to walk for much longer than ideal. This is illustrated by the following pie chart:
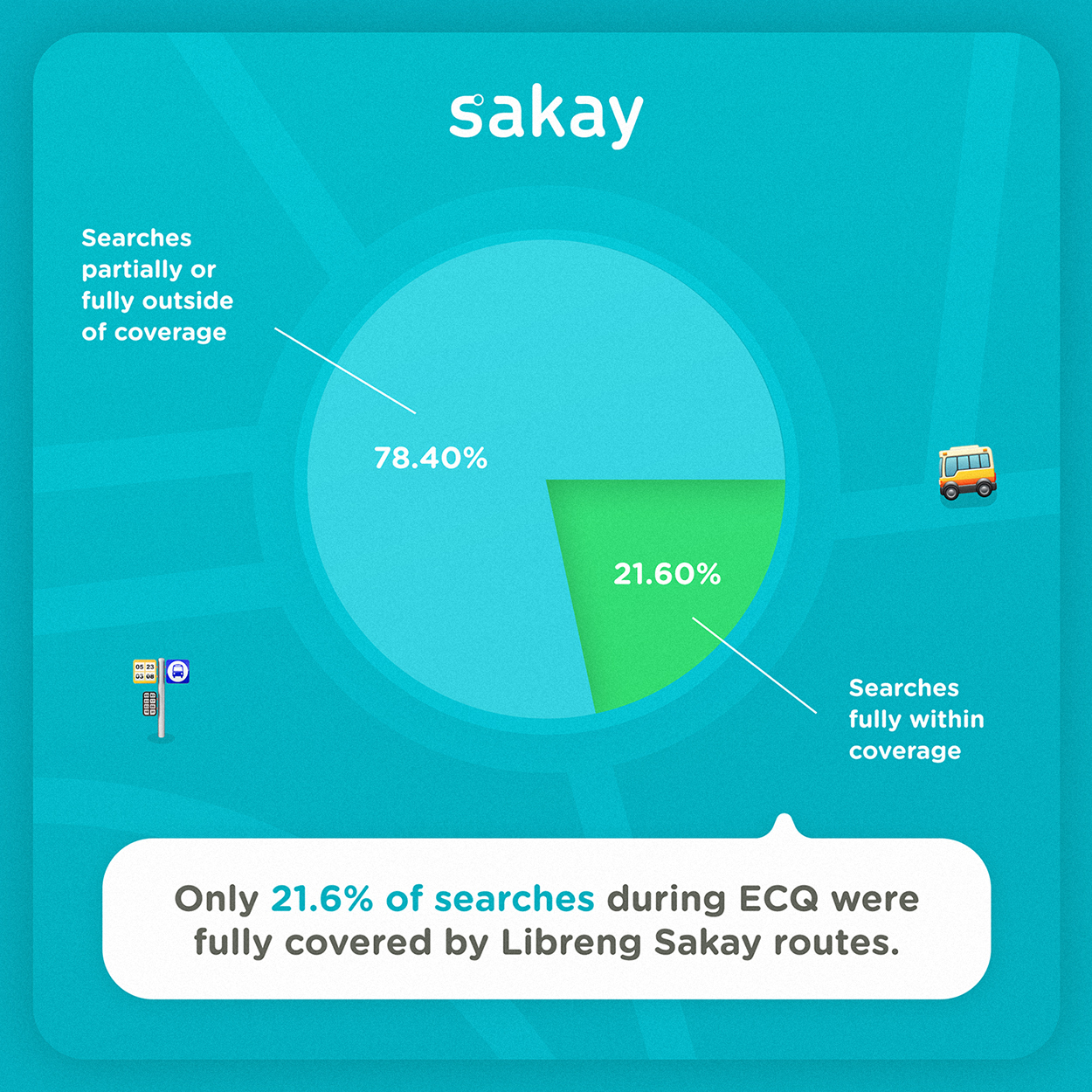
Looking exclusively at searches that fell within the coverage area, we can learn a bit more:
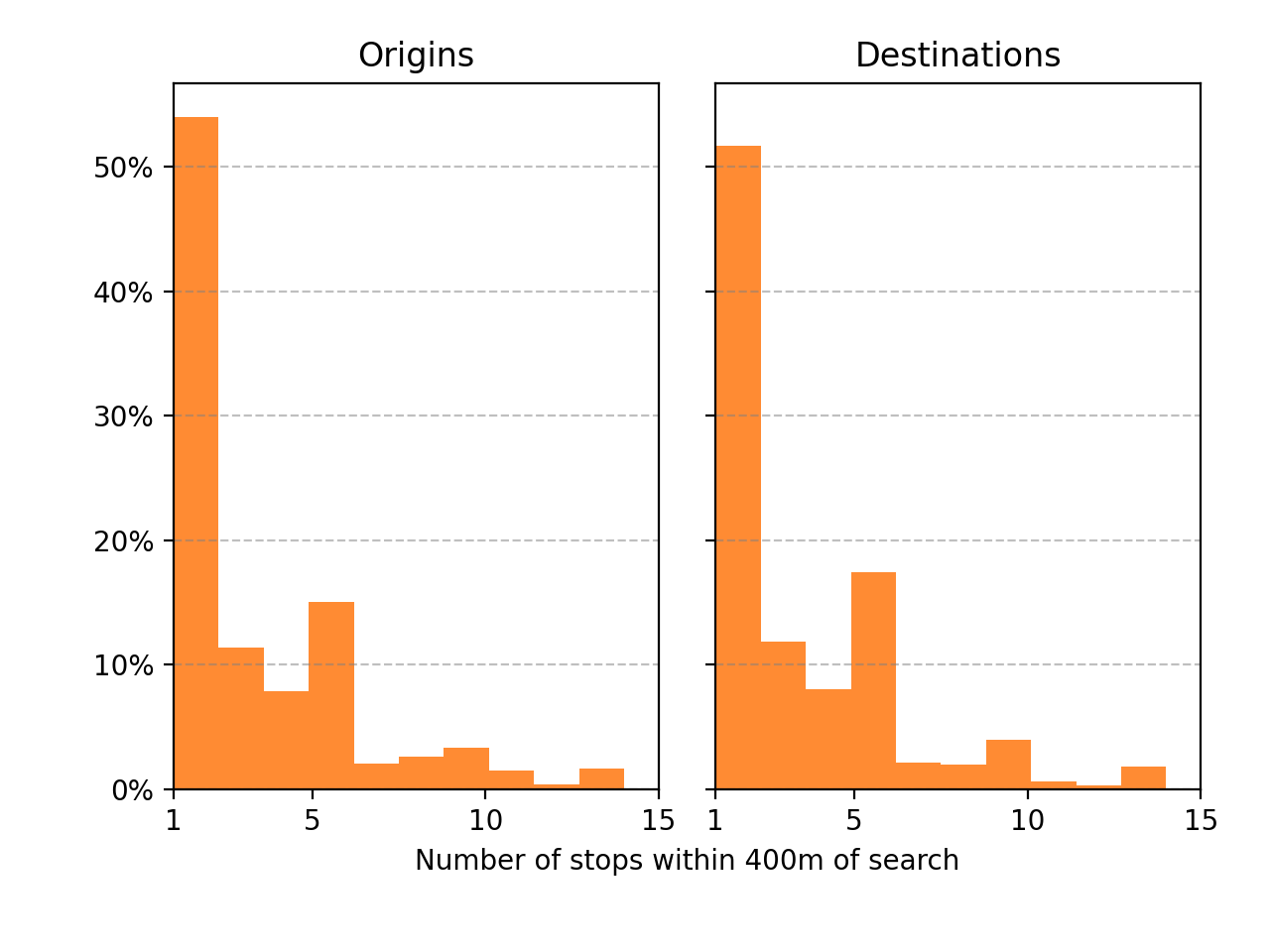
This graph shows a distribution of how many stops were accessible within 400m of walking from search origins and destinations. Note that this graph only takes into account searches with both origins and destinations within coverage.
Both graphs skew heavily to the left, meaning searches that did fall within coverage had less accessible stops. Of the search origins and destinations within coverage, more than 50% only had access to one transport stop. This is easily illustrated by the following graph, where the highest concentration of searches have 1-3 or less available stops near both the origin and destination:
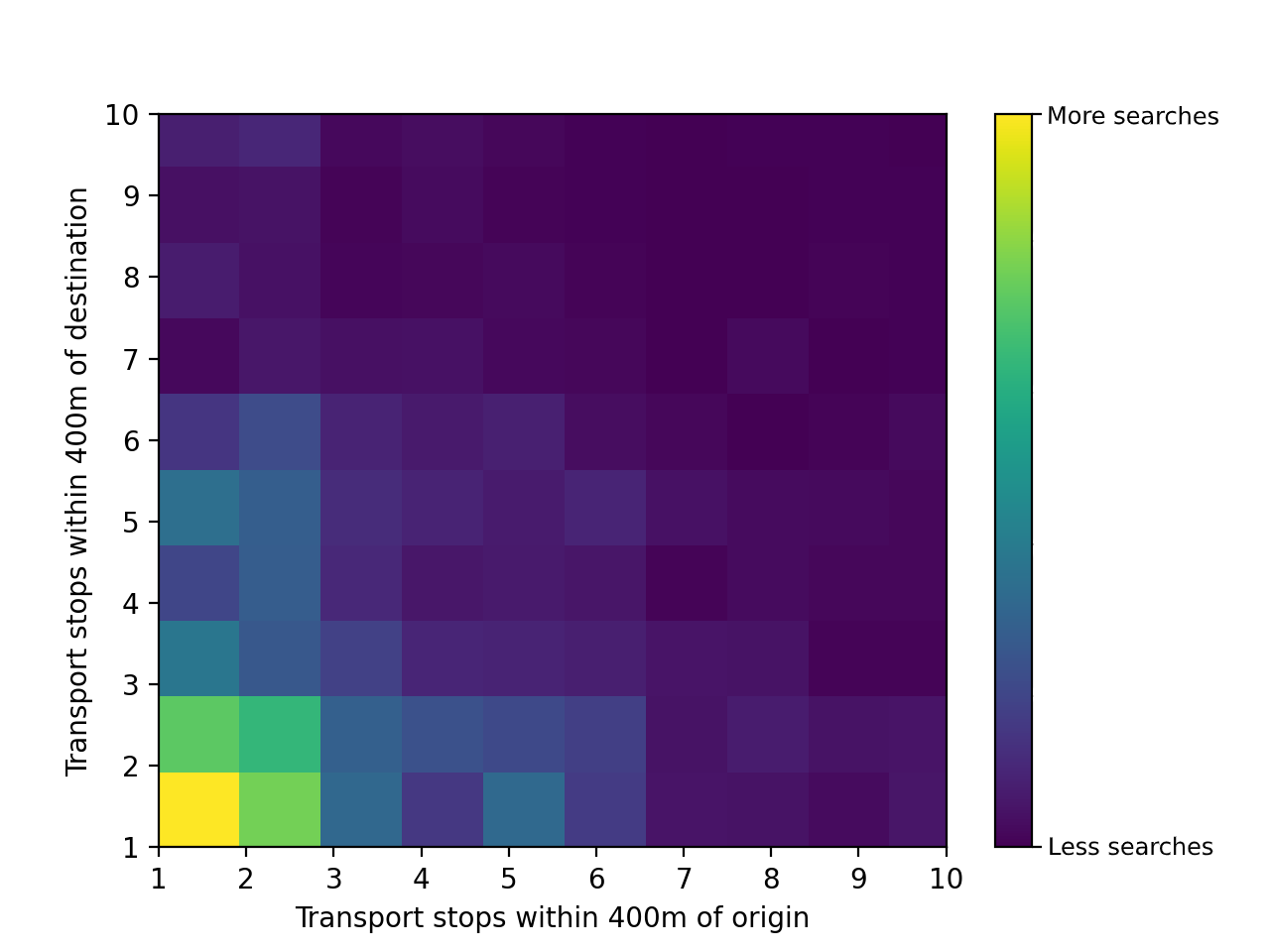
Keep in mind that the routes plied during April ECQ were often very specific or point-to-point, so there's a decent chance that searches with fewer stops available weren't served by appropriate routes. Plus, having less routes available means commuters might have to make more transfers between routes – which isn't ideal! When it comes to transport coverage, falling within walking distance of a stop isn't the only consideration. High quality coverage would also mean commuters have multiple ways to get to their destinations.
Conclusion
These kinds of demand-coverage analyses are uniquely enabled by a combination of search data from Sakay's user base and our collection of transit stop data. Our search data allows us to pinpoint where people are commuting from and where they want to go, while transit stop data helps us analyze how well that demand is being met. The case study outlined here will hopefully serve as a reference for when we need to conduct similar analyses in aid of policy or research. In the future, demand-coverage analysis could be of interest to retailers or businesses interested in analyzing consumer foot traffic, as well as government agencies looking to augment transit coverage.
Special thanks to the Department of Transportation, the Office of the Vice President, and Pasig Transport for providing frontliner routes, and helping us map them. Map data provided by OpenStreetMap contributors. Coverage map generated in kepler.gl. Connect with Matthew on LinkedIn!
Are you a student interested in geodata and public transportation? Talk to us for internships at work@sakay.ph!